This content is only available in English
Daniel Rückert - AI in Healthcare and Medicine
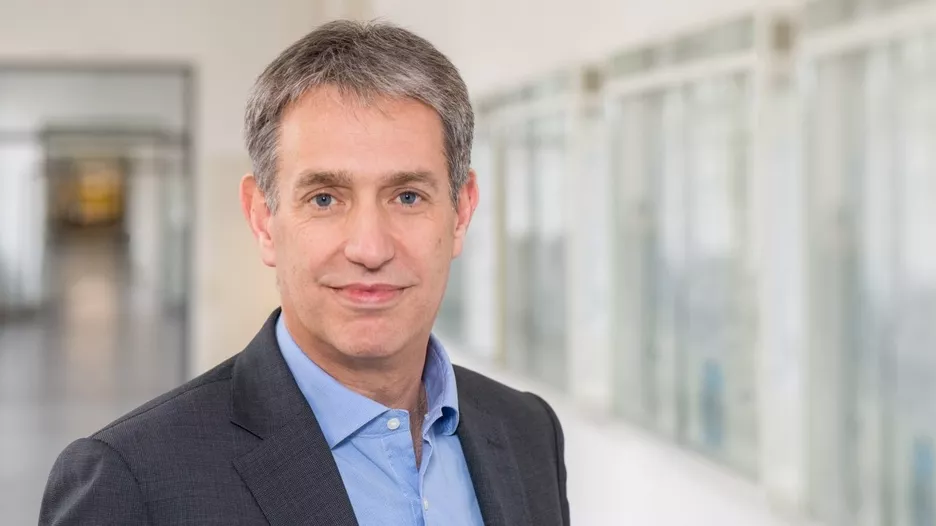
Research Focus
The Lab for AI in Medicine at TU Munich develops artificial intelligence (AI) and machine learning (ML) techniques to improve medicine for patients and healthcare professionals. Our aim is to address some of the key challenges in healthcare and medicine using AI including:
• Affordable healthcare, increased productivity of care: AI has the potential to improve clinical productivity (e.g. through smart workflows) and to reduce error rates (e.g. drug prescription).
• Prevention and early detection. This includes AI for mobile or wearable devices (digital biomarkers) as well as AI for screening (e.g. mammography).
• Diagnosis, monitoring and stratification including AI for improved diagnosis (e.g. differential diagnosis of dementia), for optimal stratification of patients for treatment as well as to monitor disease progression or health state in daily life.
• Therapy planning, treatment and interventions including AI for intelligent treatment and therapy as well as for computer-aided or robot-assisted interventions and smart assistive and/or implantable devices.
• Biomedical discovery: AI technologies can revolutionize data-driven discovery science, enabling the better understanding of diseases and their underpinning mechanisms. AI has also the potential to support the development of new interventions, treatments and drugs.
Our current research focus is on AI and ML methods for biomedical imaging including the development of innovative algorithms for biomedical image acquisition, image analysis and image interpretation – especially in the areas of image reconstruction, registration, segmentation, tracking and modelling. We are also developing AI and ML methods for extracting clinically useful information from biomedical images – especially for computer-assisted diagnosis and prognosis. Additionally, we are developing trustworthy AI methods that are explainable, transparent and privacy preserving.
We have particularly strong interest in the application of imaging and computing technology to improve the understanding brain development (in-utero and ex-utero), to improve the diagnosis and stratification of patients with dementia, stroke and traumatic brain injury as well as for the comprehensive diagnosis and management of patients with cardiovascular disease and cancer.
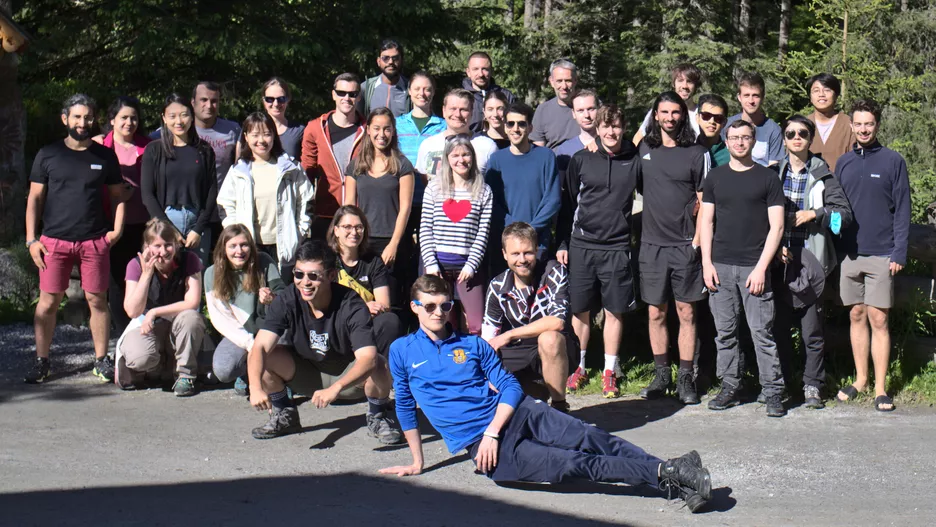
Over the last 20 years Professor Rückert has worked extensively in the area of biomedical image computing covering all aspects from image acquisition to image analysis and interpretation. His current research interests include:
- Development of algorithms for image acquisition, image analysis and image interpretation – in particular in the areas of registration, reconstruction, tracking, segmentation and modelling.
- Novel AI and machine learning algorithms for the extraction of clinically useful information from medical images – in particular for computer-aided detection, diagnosis and decision support.
Professor Rückert has a particularly strong interest in clinical translation: To facilitate translation of research to healthcare he has co-founded IXICO to commercialize the image analysis techniques developed in his research group. IXICO provides imaging analysis solutions and imaging biomarkers for clinical trials in the pharmaceutical industry and healthcare diagnostics. Since 2003, IXICO has rapidly grown and now employs 50 people. IXICO’s technologies have been used in more than 60 large-scale clinical trials involving more than 20.000 patients.
- Fellow, British Computer Society (2010)
- Fellow, MICCAI Society (2014)
- Fellow, Royal Academy of Engineering (2015)
- Fellow, IEEE (2015)
- Fellow, Academy of Medical Sciences (2019)
- Fellow, International Academy of Medical & Biological Engineering (2020)
- Alexander von Humboldt Professorship for Artificial Intelligence (2020)
- ERC Synergy Grant (2013), ERC Advanced Grant (2020)
Kaissis, G., Ziller, A., Passerat-Palmbach, J., Ryffel, T., Usynin, D., Trask, A., Lima Jr, I. Mancuso, J., Jungmann, F., Steinborn, M.-M., Saleh, A., Makowski, M., Rueckert, D. and Braren, R. (2021). End-to-end privacy preserving deep learning on multi-institutional medical imaging. Nature Machine Intelligence 3, 473–484.
Bai, W., Suzuki, H., Huang, J., Francis, C., Wang, S., Tarroni, G., Guitton, F., Aung, N., Fung, K., Petersen, S.E., Piechnik, S.K., Neubauer, S., Evangelou, E., Dehghan, A., O'Regan, D.P., Wilkins, M.R., Guo, Y., Matthews, P.M., Rueckert, D. (2020). A population-based phenome-wide association study of cardiac and aortic structure and function. Nat Med 26, 1654-1662.
Meyer, H.V., Dawes, T.J.W., Serrani, M., Bai, W., Tokarczuk, P., Cai, J., de Marvao, A., Henry, A., Lumbers, R.T., Gierten, J., Thumberger, T., Wittbrodt, J., Ware, J.S., Rueckert, D., Matthews, P.M., Prasad, S.K., Costantino, M.L., Cook, S.A., Birney, E., O'Regan, D.P. (2020). Genetic and functional insights into the fractal structure of the heart. Nature 584, 589-594.
Bello, G.A., Dawes, T.J.W., Duan, J., Biffi, C., de Marvao, A., Howard, L., Gibbs, J.S.R., Wilkins, M.R., Cook, S.A., Rueckert, D., O'Regan, D.P. (2019). Deep learning cardiac motion analysis for human survival prediction. Nat Mach Intell 1, 95-104
Qin, C., Schlemper, J., Caballero, J., Price, A.N., Hajnal, J.V., Rueckert, D. (2019). Convolutional Recurrent Neural Networks for Dynamic MR Image Reconstruction. IEEE Trans Med Imaging 38, 280-290.
Schlemper, J., Oktay, O., Schaap, M., Heinrich, M., Kainz, B., Glocker, B., Rueckert, D. (2019). Attention gated networks: Learning to leverage salient regions in medical images. Med Image Anal 53, 197-207.
Koch, L.M., Rajchl, M., Bai, W., Baumgartner, C.F., Tong, T., Passerat-Palmbach, J., Aljabar, P., Rueckert, D., Koch, L.M., Rajchl, M., Wenjia, B., Baumgartner, C.F., Tong, T., Passerat-Palmbach, J., Aljabar, P., Rueckert, D., Bai, W., Passerat-Palmbach, J., Rajchl, M., Tong, T., Baumgartner, C.F., Koch, L.M., Rueckert, D., Aljabar, P. (2018). Multi-Atlas Segmentation Using Partially Annotated Data: Methods and Annotation Strategies. IEEE Trans Pattern Anal Mach Intell 40, 1683-1696.
Parisot, S., Ktena, S.I., Ferrante, E., Lee, M., Guerrero, R., Glocker, B., Rueckert, D. (2018). Disease prediction using graph convolutional networks: Application to Autism Spectrum Disorder and Alzheimer's disease. Med Image Anal 48, 117-130.
Schlemper, J., Caballero, J., Hajnal, J.V., Price, A.N., Rueckert, D. (2018). A Deep Cascade of Convolutional Neural Networks for Dynamic MR Image Reconstruction. IEEE Trans Med Imaging 37, 491-503.
Kamnitsas, K., Ledig, C., Newcombe, V.F.J., Simpson, J.P., Kane, A.D., Menon, D.K., Rueckert, D., Glocker, B. (2017). Efficient multi-scale 3D CNN with fully connected CRF for accurate brain lesion segmentation. Med Image Anal 36, 61-78.
Prof. Dr. Daniel Rückert
Technical University of Munich
Artificial Intelligence in Healthcare and Medicine
Phone: +49 89 4140 8587
E-Mail
Assistance:
Deborah Carraro
Phone: +49 89 4140 8851
E-Mail